Across the nation, researchers, healthcare providers and public health officials are continuing to drive new population health management programs and applying new systems to support those initiatives. From an early-warning system that applies predictive analytics to improve infectious disease surveillance to an automated Machine Learning system for detecting rare and previously unseen disease clusters, population health management is making formidable strides. The following are two examples of the evolving advancements.
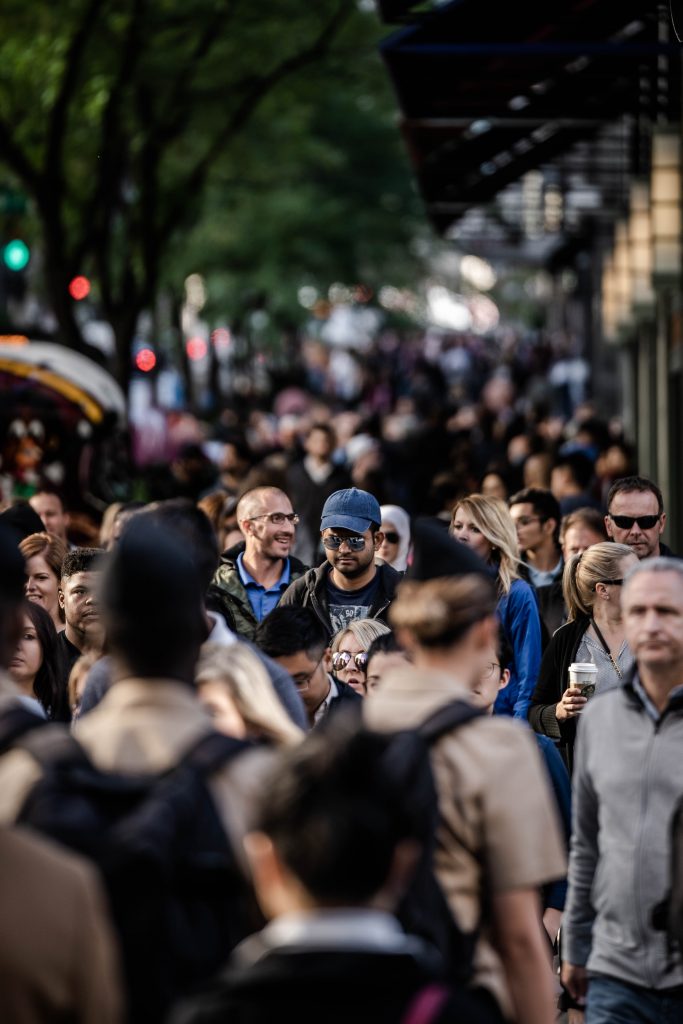
New Jersey’s New Statewide Population Health Data Project
This past June, the Rutgers Center for State Health Policy unveiled its New Jersey Integrated Population Health Data (iPHD) Project. Launched at a virtual research consortium, the project’s goal is to better inform New Jersey’s health policymaking by promoting its use of data in research. The project is also intended to help achieve a better understanding of those factors that affect population health and government program efficiency.
According to Joel Cantor, director of the Center for State Health Policy and an ex-officio member of the iPHD Governing Board, “One of the chief goals of the iPHD is to create a community of researchers in New Jersey dedicated to addressing questions about the most pressing health needs of New Jersey residents.” The iPHD will enable participating researchers to access various types of health data, including information regarding births, mortality, hospital billing records, and COVID-19 surveillance in order to study New Jersey’s population. The project’s initial priorities will be the state’s opioid epidemic, maternal and infant health, social determinants of health, COVID-19 and other public health emergencies.
Machine Learning System Now Detecting Public Health Threats
On November 4, 2022, researchers from New York University’s Machine Learning for Good Laboratory (ML4G Lab), in collaboration with researchers from Carnegie Mellon University and the New York City Department of Health and Mental Hygiene (NYC DOHMH) announced their development of an automated Machine Learning system for detecting rare or previously unseen disease clusters. Existing automated systems which detect public health threats by relying on “syndromic surveillance” to detect existing threats. They, however, are unable to effectively identify new threats.
Stated NYU Professor Daniel B. Neill, senior author of the related study and the director of the ML4G Lab, “Existing systems are good at detecting outbreaks of diseases that we already know about and are actively looking for, like flu or COVID. But what happens when something new and scary comes along? Pre-syndromic surveillance provides a safety net to identify emerging threats that other systems would fail to detect.”
The new system, known as the Multidimensional Semantic Scan (MUSES) relies on a pre-syndrome surveillance approach by leveraging digitally-communicated data on patient conditions. The researchers developed the system using anonymized chief complaint data from hospital emergency department visits and then relies on these complaints to identify trends and patterns in the words or phrases used by patients, enabling the potential detection of localized clusters.
It is likely that the attention being paid to social determinants of health, in addition to disparities in healthcare particularly relating to racial discrimination and underserved communities, will continue to drive other new and exciting population health management advancements.